HACKUTD WINNER
Project Haven
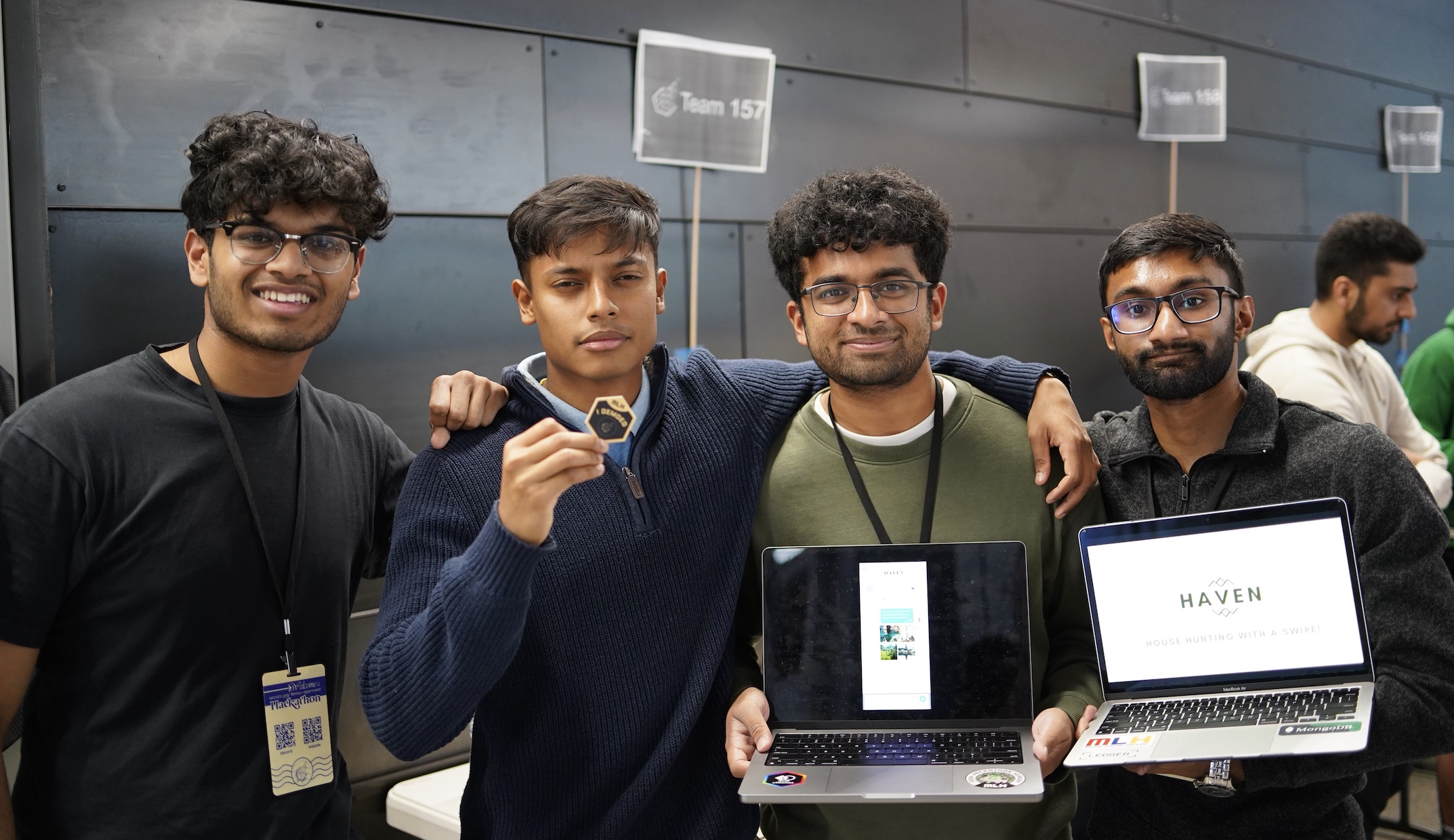


-
Hari Prasath Ramalingam
-
Sahi Sagiraju
-
Sherwin Thirumavalan
Project Acknowledgements
I would like to extend my heartfelt gratitude to Fannie Mae and HackUTD for providing us with this incredible opportunity to work on this project. Their support and resources have been invaluable throughout the process.
I would also like to thank my project team members, Hari Prasath Ramalingam, Sahishnu Sagiraju, and Sherwin Thirumavalan for their outstanding contributions, dedication, and collaboration. This project wouldn't have been possible without their hard work and shared commitment to success.
Inspiration
Inspired by the swipe-right culture of modern dating apps, we dreamed up Haven—a fun, user-friendly app where finding your dream home is as simple as finding a date. Fueled by Fannie Mae’s dedication to making housing more accessible, we’re making the whole home-buying process less daunting and a lot more personal. This is for everyone who’s ever felt lost in the sea of real estate—Haven is your lighthouse.
What it does
Homeowners: ‘Haven’ kicks off for buyers with a quick financial check-up to determine a validation score, guiding them on how ready they are to buy. Depending on the score, the app provides personalized advice to improve buying capacity. Then, it’s all about swiping through homes and fine-tuning preferences to zero in on the perfect match.
Realtors: Realtors get a dashboard to track their listings’ performance on Haven, seeing who’s interested and how buyers interact with their properties. They can prioritize and reach out to the most qualified buyers based on their scores, streamlining the connection process to quickly turn swipes into sales.
Challenges we ran into
We created a model that could predict the validation score given any value. Data Preprocessing: We started by cleaning and preparing the data, ensuring our model received high-quality input. Feature Engineering: Financial indicators like LTV, DTI, and FEDTI ratios were calculated as predictive features. Model Selection: We chose a Random Forest algorithm for its ability to handle the nuances in complex datasets. This was trained with the selected features, using the processed dataset. Testing & Evaluation: The model’s performance was later tuned for higher accuracy and reliability.
Finally, we utilized Figma to create our UI to represent the versatility in its design better.
Accomplishments that we're proud of
Our challenges were data analyzing, algorithm optimization, front-end integration, and time management. In terms of looking through the data, we had to learn terms such as LTV, FEDTI, and DTI to figure out how to create an algorithm to predict a good score. Additionally, the front end was considerably challenging to create with the limited time we were given. We switched to Figma to create a presentable UI with all the components functioning.
What's next for Haven
Moving forward, Haven is poised for growth with plans to incorporate more financial metrics into our scoring algorithm for precision-tailored advice. We aim to broaden our feature set with tools like a real-time analytics dashboard and integrated mortgage calculators to empower our users further. To enrich the home-buying journey, we’re considering gamification to boost user engagement and a community forum for shared insights and support. Scaling Haven to new markets and diversifying our user base remains a top priority, as we strive to be at the forefront of prop-tech innovation, redefining the experience of purchasing a home.